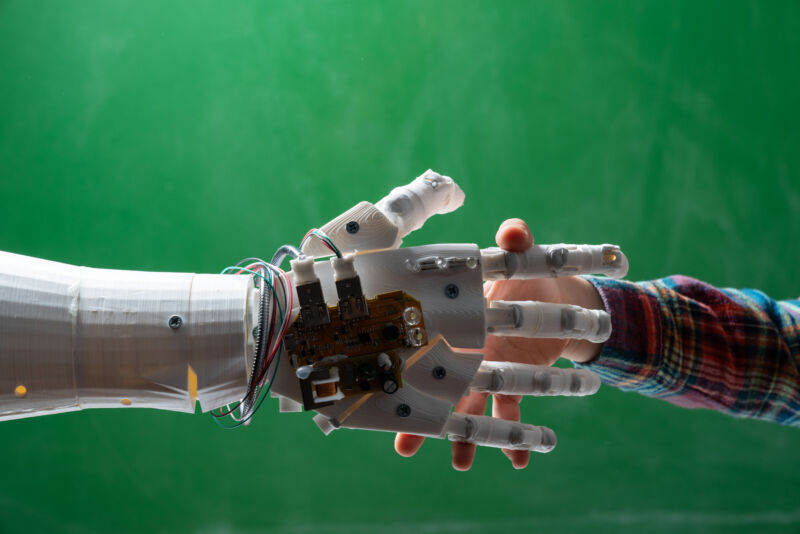
Perhaps the most famous chatbot of all time, ChatGPT parses vast amounts of text data – millions of books, articles, Wikipedia pages, and everything else its creators have found roaming the internet. By doing so, they sometimes learned human-like conversational skills.
But what if an advanced AI could learn like a small child without having to read 80 million books or see 97 million cats? Under the patient guidance of mom and dad? , you’ve just taken your first steps to explore a brave new world. A team of researchers at New York University tried it out, and it had some success.
childhood memories
“The big thing this project speaks to is the classic debate about nurture versus nature: What is built into children and what can they gain through their experiences in the outside world?” from the New York University Data Science Center. says researcher Wai Keen Vong. To find out, Vong and his team pushed AI algorithms through the closest possible approximation to human childhood. They accomplished this by populating a database called SAYCam-S. The database is filled with first-person video footage shot with a camera strapped to a baby named Sam, recording normal baby behavior from the time he was 6 to 25 years old.th One month of his life.
“In our research, we used a multimodal learning algorithm that processes visual input, such as frames from a camera and audio for children,” Vong explains. This algorithm was called Child’s View for Contrastive Learning (CVCL). It worked by converting images and words into descriptive vectors using visual and linguistic encoders. A neural network then analyzed these equations to find patterns and ultimately learned to associate the right images with the right words. (This was a common multimodal learning algorithm, not anything revolutionary.)
Based on just 61 hours of Sam’s waking hours (about 1 percent of a child’s experience), the AI learned to recognize sand, paper, puzzles, cars, and balls in images. It performed as well as standard image recognition algorithms trained the usual way through millions of examples. But I couldn’t understand hands, rooms, or baskets. Some of them didn’t click here.
incomplete slideshow
The problem was that the AI didn’t recognize Sam’s experience in the same way Sam did. Because the algorithm had access to individual frames annotated with transcribed audio, it perceived the frames more like a very long slideshow than a continuous experience. “This introduced learning artifacts,” Vong said.
For example, I struggled with the word “hand” because most of the frames included hands. Also, the time Sam’s parents used the word “hand” most often was when Sam was at the beach. Therefore, Vong explains, the AI confused “hand” with “sand.” The same applies to the word “room”. Sam spent most of his time indoors, and his parents didn’t constantly remind him that they were in the room.
Then there was the question of word frequency. Sam liked to play with balls, so he heard the word “ball” many times. However, he rarely heard the word “basketball.”
The AI couldn’t even understand the concept of movement. “All verbs have a temporal component, such as words related to movement, such as ‘push,’ ‘pull,’ and ‘twist,'” says Vong. “This is something we are actively working on as we learn from video. We already know that using video instead of still images allows for a deeper understanding of things as they unfold over time. ” he added. The next version should organize learning from continuous experience.
Driving lessons
Obviously, teaching AI to recognize balls in images has been done before. So why did Vong’s team’s work make such a splash that it was published in a science magazine rather than a second-tier AI-specific publication? It’s a possibility to build.
This is the first demonstration that AI can effectively learn from limited individual experience. It’s the difference between collecting a huge database of driving examples from hundreds of thousands of Teslas to teach an AI to drive a car, and signing up for one Tesla to take several lessons with a driving instructor. That’s the difference. The latter is easier, faster, and infinitely cheaper.
We are still far from being able to teach machines the way we teach humans. “The model we used was passive; it wasn’t designed to generate any action or provide any response on its own,” Vong says.
Still, there is a lot of room for improvement in this system. These include using a database that takes up more than 1 percent of the child’s time and adding information other than text and images (which may include sounds, smells, tactile sensations, emotional charge, etc.). “But all of this can be achieved by extending the AI we already have, rather than starting from scratch,” Vong argues.
This suggests that we are much less special than we think. “Whether driving or learning a language, humans are much more sample efficient than AI. A big part of our job is figuring out what makes them more sample efficient and how we can use that to create smarter It’s about understanding what you’re building the machine for,” Vong says.
Jacek Krywko is a science and technology writer based in Olsztyn, Poland. He covers research in space exploration and artificial intelligence.